Default Loan Prediction Based On Customer Behavior Using Machine Learning And

Default loan prediction is a critical task for financial institutions. The ability to accurately predict which customers are likely to default on their loans can help banks and other lenders make better lending decisions, reduce their risk of loss, and improve their profitability.
4.8 out of 5
Language | : | English |
File size | : | 2502 KB |
Text-to-Speech | : | Enabled |
Screen Reader | : | Supported |
Enhanced typesetting | : | Enabled |
Print length | : | 197 pages |
Lending | : | Enabled |
In recent years, machine learning and data mining techniques have been increasingly used to develop default loan prediction models. These techniques allow lenders to analyze large amounts of data, identify patterns and relationships, and build predictive models that can be used to assess the risk of default for individual customers.
There are a number of different machine learning and data mining techniques that can be used for default loan prediction. Some of the most commonly used techniques include:
- Logistic regression
- Decision trees
- Support vector machines
- Neural networks
- Ensemble methods
The choice of which machine learning technique to use for default loan prediction depends on a number of factors, including the size and quality of the data available, the desired level of accuracy, and the computational resources available.
In general, the more data that is available, the more complex the machine learning model that can be used. However, more complex models also require more computational resources to train and deploy.
The desired level of accuracy is also an important consideration. For some applications, a simple model that is able to achieve a high level of accuracy may be sufficient. However, for other applications, a more complex model that is able to achieve a very high level of accuracy may be necessary.
The computational resources available are also a factor to consider. Some machine learning techniques require significant computational resources to train and deploy, while others require very little.
Once a machine learning model has been developed, it can be used to score new customers and assess their risk of default. The score is a number that represents the probability that the customer will default on their loan.
Lenders can use the score to make lending decisions. For example, they may approve loans for customers with low scores and deny loans for customers with high scores.
Default loan prediction models can help lenders make better lending decisions, reduce their risk of loss, and improve their profitability. However, it is important to note that these models are not perfect. There is always a risk that a customer will default on their loan, even if they have a low score.
Lenders should therefore use default loan prediction models in conjunction with other information, such as the customer's credit history and financial situation, when making lending decisions.
Default loan prediction is a critical task for financial institutions. Machine learning and data mining techniques can be used to develop default loan prediction models that can help lenders make better lending decisions, reduce their risk of loss, and improve their profitability.
4.8 out of 5
Language | : | English |
File size | : | 2502 KB |
Text-to-Speech | : | Enabled |
Screen Reader | : | Supported |
Enhanced typesetting | : | Enabled |
Print length | : | 197 pages |
Lending | : | Enabled |
Do you want to contribute by writing guest posts on this blog?
Please contact us and send us a resume of previous articles that you have written.
Book
Novel
Page
Chapter
Text
Story
Reader
Paperback
E-book
Magazine
Newspaper
Bookmark
Shelf
Foreword
Scroll
Tome
Bestseller
Narrative
Biography
Encyclopedia
Dictionary
Thesaurus
Narrator
Librarian
Stacks
Periodicals
Lending
Reserve
Academic
Journals
Special Collections
Interlibrary
Literacy
Study Group
Thesis
Dissertation
Storytelling
Reading List
Book Club
Theory
Rainer Maria Rilke
David P Redlawsk
Sara Herranz
Randy Charles Epping
John Braddock
Megan Kreiner
Amali Gunasekera
Lynn Michelsohn
Lance Marcum
Chris Hall
Murray Grodner
Michelle Richmond
Sally Dixon
Matthew A Barsalou
Reginald Leon Green
Pamela Hanlon
Ann Lewin Benham
Hda Roberts
Paul W Papa
Mary Renault
Light bulbAdvertise smarter! Our strategic ad space ensures maximum exposure. Reserve your spot today!
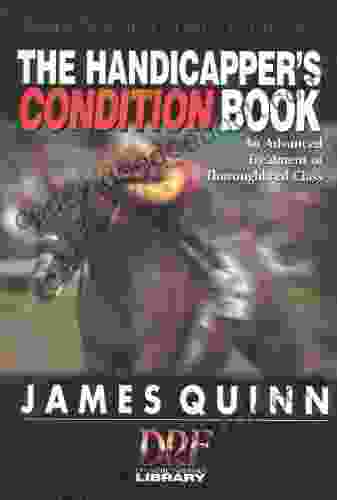

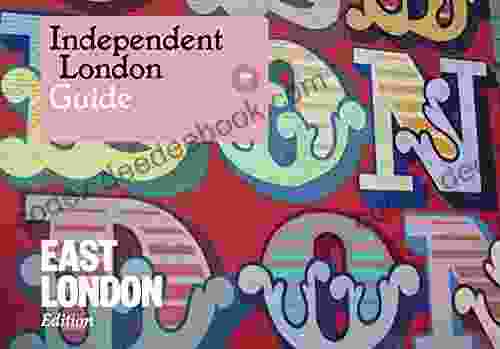

- Samuel Taylor ColeridgeFollow ·17.5k
- Chase MorrisFollow ·6.2k
- Derrick HughesFollow ·4.8k
- Hugo CoxFollow ·19.4k
- Marcel ProustFollow ·3.4k
- Pablo NerudaFollow ·19.8k
- George R.R. MartinFollow ·5k
- Gerald BellFollow ·9.7k
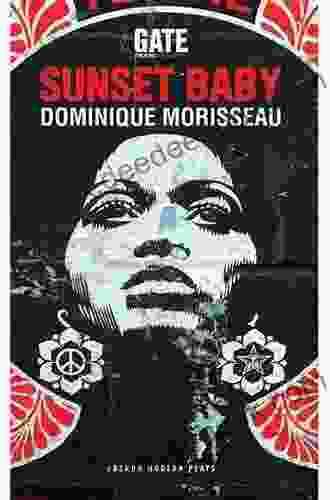

Sunset Baby Oberon: A Riveting Exploration of Modern...
In the realm of...
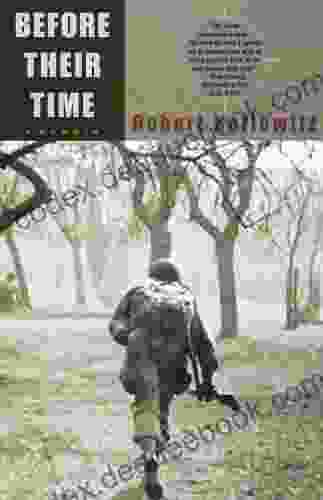

Before Their Time: A Memoir of Loss and Hope for Parents...
Losing a child is a tragedy...
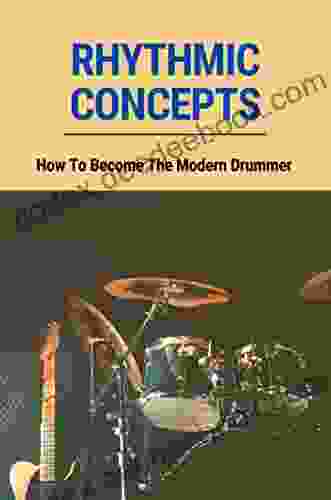

Rhythmic Concepts: How to Become the Modern Drummer
In the ever-evolving...
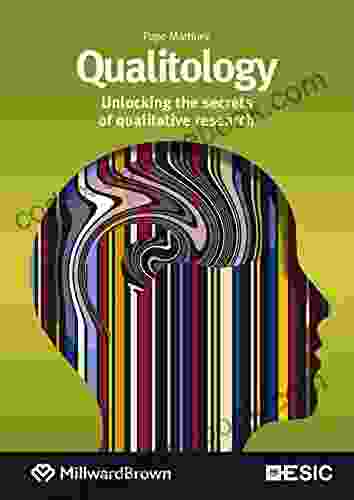

Qualitology: Unlocking the Secrets of Qualitative...
Qualitative research is a...
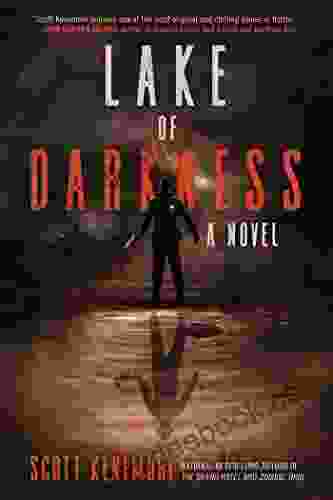

Unveiling the Secrets of the Lake of Darkness Novel: A...
A Journey into Darkness...
4.8 out of 5
Language | : | English |
File size | : | 2502 KB |
Text-to-Speech | : | Enabled |
Screen Reader | : | Supported |
Enhanced typesetting | : | Enabled |
Print length | : | 197 pages |
Lending | : | Enabled |