Online Retail Clustering and Prediction Using Machine Learning with Python GUI

The online retail industry is booming, with more and more consumers choosing to purchase products and services online. This has led to a wealth of data being generated about customer behavior, which can be used to improve the customer experience and increase sales. One way to use this data is to apply machine learning techniques to cluster customers into different segments and predict their future purchases.
In this article, we will explore how to use machine learning techniques to cluster and predict customer behavior in the online retail domain. We will use a real-world dataset and Python to build a machine learning model that can identify customer segments and predict their future purchases. The model will be deployed using a user-friendly graphical user interface (GUI) that allows users to interact with the model and make predictions.
4.7 out of 5
Language | : | English |
File size | : | 287 KB |
Text-to-Speech | : | Enabled |
Screen Reader | : | Supported |
Enhanced typesetting | : | Enabled |
Word Wise | : | Enabled |
Print length | : | 42 pages |
Lending | : | Enabled |
Clustering Customer Data
The first step in building a machine learning model for online retail is to cluster the customer data into different segments. This can be done using a variety of clustering algorithms, such as k-means or hierarchical clustering. The goal of clustering is to identify groups of customers who have similar characteristics, such as buying habits or demographics.
Once the customer data has been clustered, we can use the cluster labels to identify the different customer segments. For example, we might identify a segment of customers who are likely to purchase high-value items, or a segment of customers who are likely to churn. This information can be used to develop targeted marketing campaigns and improve the customer experience.
Predicting Customer Purchases
Once we have identified the different customer segments, we can use machine learning techniques to predict their future purchases. This can be done using a variety of predictive modeling algorithms, such as logistic regression or decision trees. The goal of predictive modeling is to build a model that can predict the likelihood that a customer will purchase a particular product or service.
Once the predictive model has been built, we can use it to score new customers and identify those who are most likely to make a purchase. This information can be used to target marketing campaigns and improve the customer experience.
Deploying the Machine Learning Model
Once the machine learning model has been built, we need to deploy it so that it can be used by end users. One way to deploy a machine learning model is to use a graphical user interface (GUI). A GUI allows users to interact with the model and make predictions without having to write any code.
In this article, we will use the Python Tkinter library to build a GUI for our machine learning model. Tkinter is a cross-platform GUI library that is included with Python. The GUI will allow users to load the customer data, select the clustering algorithm, and make predictions.
In this article, we have explored how to use machine learning techniques to cluster and predict customer behavior in the online retail domain. We have used a real-world dataset and Python to build a machine learning model that can identify customer segments and predict their future purchases. The model has been deployed using a user-friendly graphical user interface (GUI) that allows users to interact with the model and make predictions.
The techniques described in this article can be used to improve the customer experience and increase sales in the online retail industry. By understanding the different customer segments and predicting their future purchases, businesses can develop targeted marketing campaigns and improve the customer experience.
Additional Resources
- Scikit-learn: A Python library for machine learning.
- Tkinter: A Python library for building graphical user interfaces.
- Online Retail Dataset: A real-world dataset of online retail transactions.
4.7 out of 5
Language | : | English |
File size | : | 287 KB |
Text-to-Speech | : | Enabled |
Screen Reader | : | Supported |
Enhanced typesetting | : | Enabled |
Word Wise | : | Enabled |
Print length | : | 42 pages |
Lending | : | Enabled |
Do you want to contribute by writing guest posts on this blog?
Please contact us and send us a resume of previous articles that you have written.
Book
Page
Chapter
Text
Genre
Reader
Library
Magazine
Paragraph
Shelf
Glossary
Bibliography
Preface
Footnote
Manuscript
Codex
Tome
Narrative
Biography
Encyclopedia
Dictionary
Narrator
Resolution
Librarian
Catalog
Card Catalog
Borrowing
Stacks
Study
Scholarly
Lending
Academic
Reading Room
Interlibrary
Study Group
Thesis
Awards
Reading List
Theory
Textbooks
James Quinn
Michelle Steinke Baumgard
Konrad Maurer
Fiona Mcgillivray
Kate Alcott
Matt Dobkin
Shelly Field
Paul W Papa
Earle B Young
Greg Pincus
Marilyn Waring
Cheryl Fall
H R Conklin
Jerry Craven
Jayne Peace Pyle
Charles Pidgeon
Maxim D Shrayer
Desiree
Heather B Macintosh
Jeannie Chambers
Light bulbAdvertise smarter! Our strategic ad space ensures maximum exposure. Reserve your spot today!
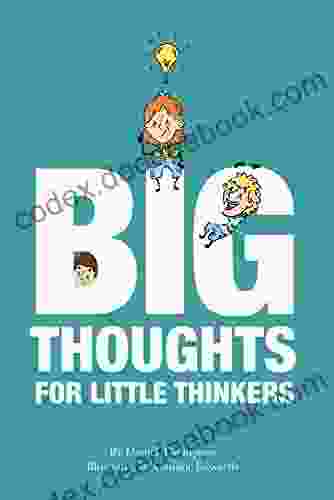

- Matt ReedFollow ·16.2k
- Ralph EllisonFollow ·10.7k
- Dennis HayesFollow ·4.1k
- Mason PowellFollow ·6.3k
- Arthur C. ClarkeFollow ·19.7k
- Richard SimmonsFollow ·16.1k
- Joe SimmonsFollow ·4.7k
- Dan BrownFollow ·7.4k
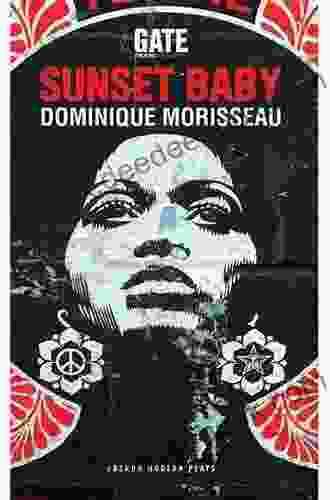

Sunset Baby Oberon: A Riveting Exploration of Modern...
In the realm of...
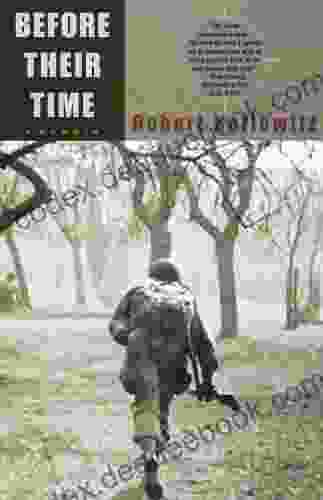

Before Their Time: A Memoir of Loss and Hope for Parents...
Losing a child is a tragedy...
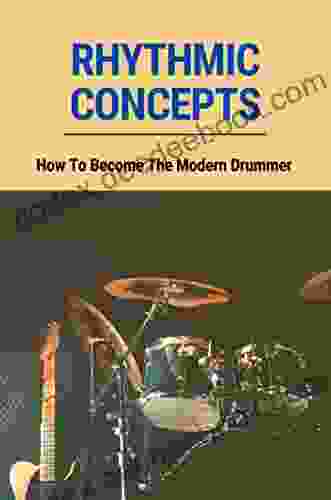

Rhythmic Concepts: How to Become the Modern Drummer
In the ever-evolving...
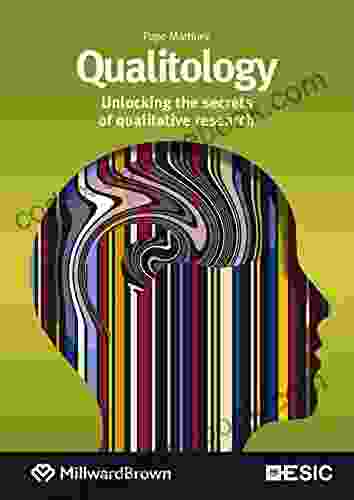

Qualitology: Unlocking the Secrets of Qualitative...
Qualitative research is a...
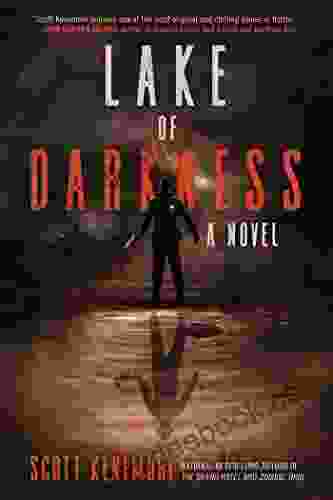

Unveiling the Secrets of the Lake of Darkness Novel: A...
A Journey into Darkness...
4.7 out of 5
Language | : | English |
File size | : | 287 KB |
Text-to-Speech | : | Enabled |
Screen Reader | : | Supported |
Enhanced typesetting | : | Enabled |
Word Wise | : | Enabled |
Print length | : | 42 pages |
Lending | : | Enabled |