Powerful Integration of Data Science and Process Engineering: A Continuous Path to Optimized Outcomes

:
In the rapidly evolving landscape of industrial operations, the convergence of data science and process engineering has emerged as a game-changer. This potent integration unlocks a treasure trove of opportunities to enhance process efficiency, drive innovation, and achieve unparalleled levels of performance.
5 out of 5
Language | : | English |
File size | : | 5272 KB |
Screen Reader | : | Supported |
Print length | : | 142 pages |
Data Science: A Catalyst for Process Insight
Data science empowers process engineers with a robust toolkit to extract meaningful insights from vast amounts of data. Advanced statistical techniques, machine learning algorithms, and artificial intelligence (AI) enable the identification of patterns, correlations, and anomalies that would otherwise remain obscured.
By harnessing the power of data science, process engineers can gain a comprehensive understanding of:
- Process variables and their interactions
- Equipment performance and degradation patterns
- Yield and quality fluctuations
Process Engineering: Laying the Foundation for Data-Driven Optimization
Process engineering provides the essential foundation for implementing data-driven optimization strategies. Engineers possess a deep understanding of process dynamics, equipment capabilities, and safety constraints. This knowledge enables them to design and implement data collection systems, develop process models, and translate insights into actionable improvements.
Continuous Improvement: The Enduring Cycle
The integration of data science and process engineering establishes a continuous improvement cycle that drives constant refinement and optimization. The process unfolds as follows:
- Data Collection and Analysis: Data is continuously collected from sensors, historians, and other sources. Data science techniques are employed to analyze the data, uncover trends, and identify anomalies.
- Process Modeling and Simulation: Process engineers develop models that simulate the process behavior based on the insights gained from data analysis. These models allow for testing different operating conditions and scenarios without disrupting actual operations.
- Optimization and Implementation: Using the insights from process modeling and simulation, engineers optimize process parameters and implement improvements. These improvements can range from minor adjustments to major overhauls.
- Performance Monitoring and Evaluation: Post-implementation, performance is closely monitored and evaluated. The results are analyzed using data science techniques to assess the effectiveness of the improvements and identify areas for further optimization.
Benefits of Data Science and Process Engineering Integration:
The integration of data science and process engineering brings forth a multitude of benefits, including:
- Increased Process Efficiency: Optimized process parameters, coupled with predictive maintenance, lead to reduced downtime, improved throughput, and enhanced resource utilization.
- Enhanced Product Quality: Real-time monitoring and control systems, powered by data science, help maintain consistent product quality, reduce defects, and meet stringent specifications.
- Reduced Operating Costs: Predictive analytics and optimization techniques identify areas for energy savings, raw material efficiency, and waste reduction, resulting in significant cost savings.
- Improved Safety and Compliance: Data science algorithms can detect anomalies and predict potential safety hazards, enabling proactive measures to mitigate risks and ensure compliance with industry regulations.
- Accelerated Innovation: The continuous flow of data and insights fosters a culture of innovation, allowing engineers to explore new process designs, materials, and technologies with greater confidence.
Case Studies: Examples of Success
Numerous case studies demonstrate the transformative impact of integrating data science and process engineering:
- Chemical Manufacturing: Data science algorithms were used to optimize the blending process in a chemical plant, resulting in a 15% increase in product yield.
- Pharmaceutical Production: Predictive analytics models were implemented to forecast equipment failures in a pharmaceutical manufacturing facility, reducing downtime by 30%.
- Pulp and Paper Industry: Data-driven process control systems improved the efficiency of a paper machine, reducing energy consumption by 10% and increasing production capacity by 5%.
:
The integration of data science and process engineering represents a paradigm shift in the optimization of industrial processes. By harnessing the power of data and advanced analytical techniques, process engineers can unlock unprecedented levels of insight, efficiency, and continuous improvement. This transformative fusion is poised to revolutionize the way industries operate, leading to enhanced competitiveness, sustainability, and innovation in the years to come.
As technology continues to advance, the convergence of data science and process engineering will only deepen, membuka jalan baru opportunities for process optimization and delivering exceptional outcomes.
5 out of 5
Language | : | English |
File size | : | 5272 KB |
Screen Reader | : | Supported |
Print length | : | 142 pages |
Do you want to contribute by writing guest posts on this blog?
Please contact us and send us a resume of previous articles that you have written.
Book
Novel
Page
Chapter
Text
Story
Genre
E-book
Magazine
Sentence
Bookmark
Glossary
Bibliography
Foreword
Synopsis
Annotation
Scroll
Codex
Biography
Memoir
Reference
Encyclopedia
Dictionary
Narrator
Resolution
Librarian
Catalog
Borrowing
Archives
Study
Lending
Reserve
Academic
Journals
Reading Room
Literacy
Study Group
Storytelling
Awards
Reading List
Conrad Black
Christopher Dyment
Michael W Mcconnell
Konrad Maurer
Shen Lee
Anita Brearton
Gail Honeyman
Ian Shapiro
Michelle Richmond
Jacie Middlemann
Jan Patek
Alphabet Rockers
Kelly Wiese
Frances Bloxam
Mary Renault
Collette Scott
Karen Brody
Bill Adler
Brahim Aouinat
Pascale Joannin
Light bulbAdvertise smarter! Our strategic ad space ensures maximum exposure. Reserve your spot today!
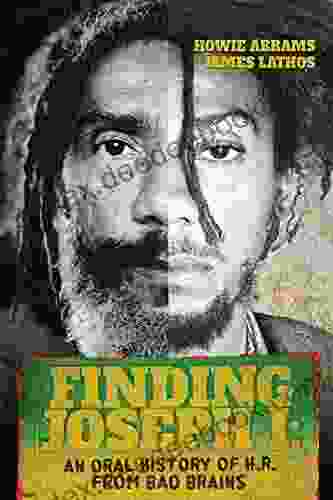

- Deacon BellFollow ·2.2k
- Rex HayesFollow ·19.8k
- Jack PowellFollow ·4.4k
- Simon MitchellFollow ·14.7k
- Devon MitchellFollow ·17.9k
- Brennan BlairFollow ·16.2k
- Robert BrowningFollow ·6k
- Sean TurnerFollow ·12k
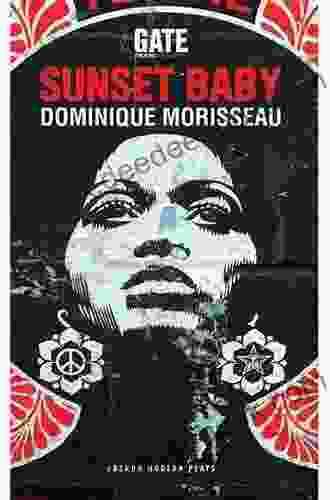

Sunset Baby Oberon: A Riveting Exploration of Modern...
In the realm of...
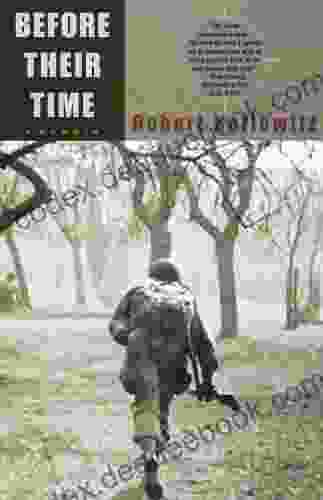

Before Their Time: A Memoir of Loss and Hope for Parents...
Losing a child is a tragedy...
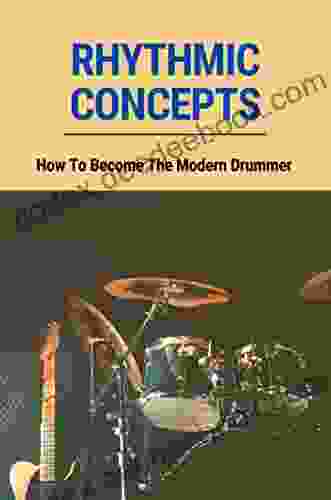

Rhythmic Concepts: How to Become the Modern Drummer
In the ever-evolving...
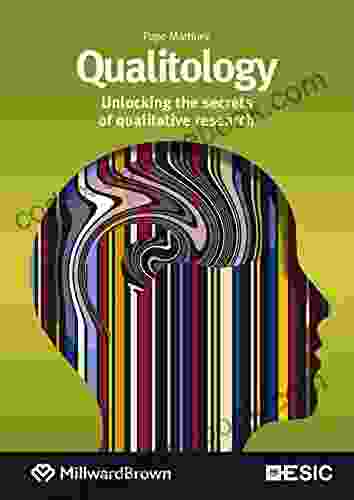

Qualitology: Unlocking the Secrets of Qualitative...
Qualitative research is a...
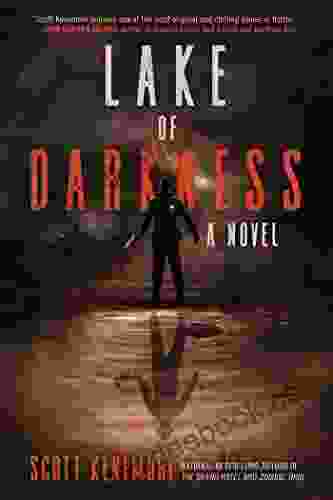

Unveiling the Secrets of the Lake of Darkness Novel: A...
A Journey into Darkness...
5 out of 5
Language | : | English |
File size | : | 5272 KB |
Screen Reader | : | Supported |
Print length | : | 142 pages |