Hands-On Neural Networks with Keras: A Comprehensive Guide

Neural networks are a powerful type of machine learning model that can be used to solve a wide variety of problems, from image classification to natural language processing. Keras is a popular deep learning library that makes it easy to build and train neural networks. In this guide, we will provide a comprehensive overview of neural networks and Keras, and we will walk through several hands-on examples of how to use Keras to build and train neural networks.
Neural networks are inspired by the human brain, and they are designed to learn from data in a similar way that the human brain learns from experience. Neural networks consist of layers of interconnected nodes, or neurons. Each neuron takes in a set of inputs, and it produces an output. The output of each neuron is then passed to the next layer of neurons, and so on.
The first layer of a neural network is called the input layer. The input layer takes in the raw data that the network is going to learn from. The last layer of a neural network is called the output layer. The output layer produces the final output of the network.
4.1 out of 5
Language | : | English |
File size | : | 19568 KB |
Text-to-Speech | : | Enabled |
Screen Reader | : | Supported |
Enhanced typesetting | : | Enabled |
Print length | : | 811 pages |
The layers between the input layer and the output layer are called hidden layers. Hidden layers allow the network to learn complex relationships between the input data and the output data.
Keras is a deep learning library that makes it easy to build and train neural networks. Keras is written in Python, and it is compatible with TensorFlow, a popular deep learning framework. Keras provides a high-level API that makes it easy to create and train neural networks, even if you don't have a lot of experience with deep learning.
In this section, we will walk through several hands-on examples of how to use Keras to build and train neural networks.
Image Classification
In this example, we will use Keras to build and train a neural network to classify images. We will use the MNIST dataset, which consists of 70,000 handwritten digits.
To get started, we need to import the necessary libraries.
python import tensorflow as tf from tensorflow.keras import datasets, models, layers
Next, we need to load the MNIST dataset.
python (train_images, train_labels),(test_images, test_labels) = datasets.mnist.load_data()
The MNIST dataset is divided into a training set and a test set. The training set contains 60,000 images, and the test set contains 10,000 images.
Next, we need to preprocess the data. We need to normalize the pixel values of the images so that they are between 0 and 1.
python train_images = train_images / 255.0 test_images = test_images / 255.0
Now we can build the neural network. We will use a simple convolutional neural network (CNN).
python model = models.Sequential([ layers.Conv2D(32, (3, 3),activation='relu', input_shape=(28, 28, 1)),layers.MaxPooling2D((2, 2)),layers.Conv2D(64, (3, 3),activation='relu'),layers.MaxPooling2D((2, 2)),layers.Flatten(),layers.Dense(128, activation='relu'),layers.Dense(10, activation='softmax') ])
The CNN consists of a stack of convolutional layers and pooling layers. The convolutional layers learn to extract features from the images, and the pooling layers reduce the dimensionality of the data. The final fully connected layers are used to classify the images.
Next, we need to compile the model. We will use the Adam optimizer and the sparse categorical cross-entropy loss function.
python model.compile(optimizer='adam', loss='sparse_categorical_crossentropy', metrics=['accuracy'])
Finally, we can train the model.
python model.fit(train_images, train_labels, epochs=10)
The model will train for 10 epochs. After each epoch, the model will evaluate its accuracy on the test set.
Once the model has finished training, we can evaluate its accuracy on the test set.
python test_loss, test_acc = model.evaluate(test_images, test_labels, verbose=2) print('\nTest accuracy:', test_acc)
The model should achieve an accuracy of over 95% on the test set.
Natural Language Processing
In this example, we will use Keras to build and train a neural network to classify text. We will use the Reuters dataset, which consists of over 10,000 news articles.
To get started, we need to import the necessary libraries.
python import tensorflow as tf from tensorflow.keras import datasets, models, layers
Next, we need to load the Reuters dataset.
python (train_data, train_labels),(test_data, test_labels) = datasets.reuters.load_data()
The Reuters dataset is divided into a training set and a test set. The training set contains 8,982 news articles, and the test set contains 2,246 news articles.
Next, we need to preprocess the data. We need to tokenize the news articles and convert them into numerical sequences.
python from tensorflow.keras.preprocessing.text import Tokenizer
tokenizer = Tokenizer(num_words=10000) tokenizer.fit_on_texts(train_data)
train_sequences = tokenizer.texts_to_sequences(train_data) test_sequences = tokenizer.texts_to_sequences(test_data)
Now we can build the neural network. We will use a simple recurrent neural network (RNN).
python model = models.Sequential([ layers.Embedding(10000, 128),layers.LSTM(128),layers.Dense(46, activation='softmax') ])
The RNN consists of an embedding layer, an LSTM layer, and a fully connected layer. The embedding layer converts the numerical sequences into dense vectors. The LSTM layer learns to extract features from the sequences, and the fully connected layer is used to classify the sequences.
Next, we need to compile the model. We will use the Adam optimizer and the sparse categorical cross-entropy loss function.
python model.compile(optimizer='adam', loss='sparse_categorical_crossentropy', metrics=['accuracy'])
Finally, we can train the model.
python model.fit(train_sequences, train_labels, epochs=10)
The model will train for 10 epochs. After each epoch, the model will evaluate its accuracy on the test set.
Once the model has finished training, we can evaluate its accuracy on the test set.
python test_loss, test_acc = model.evaluate(test_sequences, test_labels, verbose=2) print('\nTest accuracy:', test_acc)
The model should achieve an accuracy of over 90% on the test set.
In this guide, we have provided a comprehensive overview of neural networks and Keras. We have also walked through several hands-on examples of how to use Keras to build and train neural networks. We encourage you to experiment with Keras and to explore the many different ways that neural networks can be used to solve real-world problems.
4.1 out of 5
Language | : | English |
File size | : | 19568 KB |
Text-to-Speech | : | Enabled |
Screen Reader | : | Supported |
Enhanced typesetting | : | Enabled |
Print length | : | 811 pages |
Do you want to contribute by writing guest posts on this blog?
Please contact us and send us a resume of previous articles that you have written.
Book
Page
Text
Genre
Paperback
E-book
Magazine
Newspaper
Sentence
Bookmark
Glossary
Preface
Synopsis
Annotation
Manuscript
Scroll
Codex
Classics
Narrative
Biography
Autobiography
Encyclopedia
Thesaurus
Narrator
Character
Resolution
Borrowing
Stacks
Archives
Periodicals
Academic
Journals
Reading Room
Special Collections
Interlibrary
Literacy
Thesis
Dissertation
Storytelling
Theory
Mark West
Paz Oshran
Bruce Bryans
Alyson Richman
Sam Dogra
Nirvana Blaque
H H Lee
Lesley Parr
Roya Akhavan Ph D
Chalmers Brothers
Julia London
Myriem Bouzaher
Sara Herranz
Jerry Craven
P C Zick
Neil Firth
Benjamin Silliman
Philip N Howard
S E Burr
Michael Hoffman
Light bulbAdvertise smarter! Our strategic ad space ensures maximum exposure. Reserve your spot today!
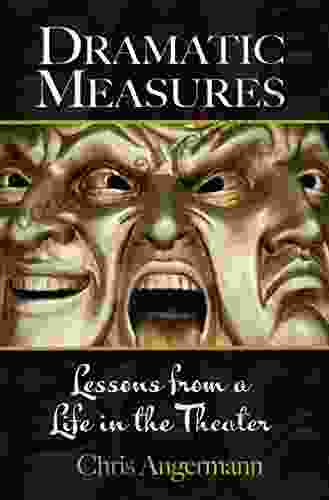

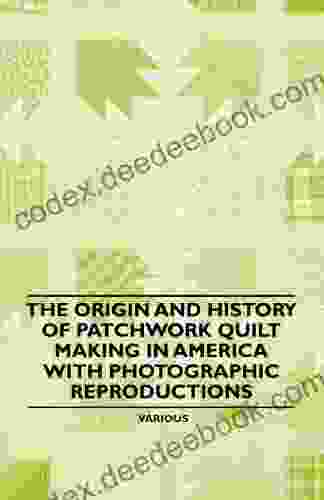

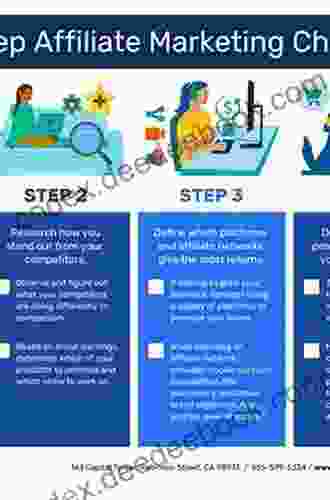

- Frank MitchellFollow ·6.8k
- Andrew BellFollow ·15.3k
- Warren BellFollow ·9.3k
- Mason PowellFollow ·6.3k
- Roland HayesFollow ·8.9k
- Richard WrightFollow ·18.6k
- Corbin PowellFollow ·5.2k
- Dan BellFollow ·19.2k
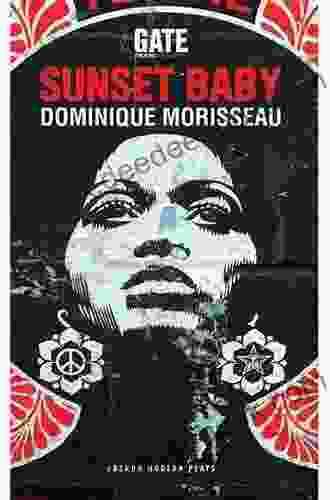

Sunset Baby Oberon: A Riveting Exploration of Modern...
In the realm of...
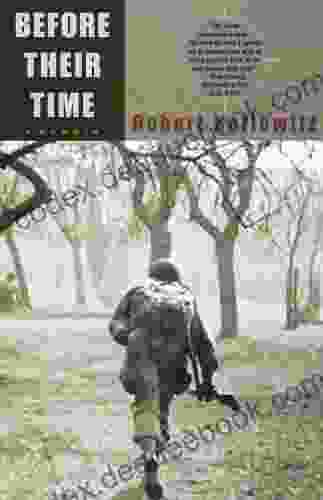

Before Their Time: A Memoir of Loss and Hope for Parents...
Losing a child is a tragedy...
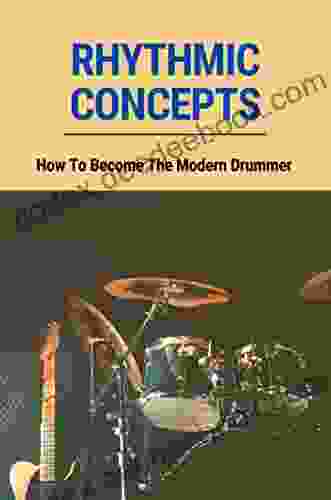

Rhythmic Concepts: How to Become the Modern Drummer
In the ever-evolving...
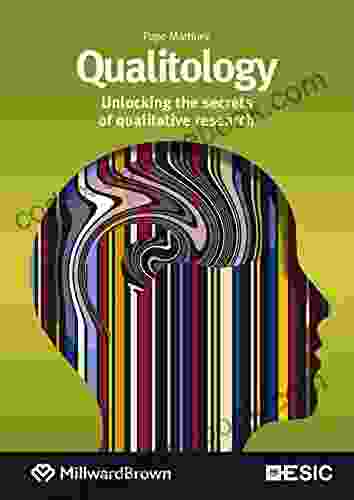

Qualitology: Unlocking the Secrets of Qualitative...
Qualitative research is a...
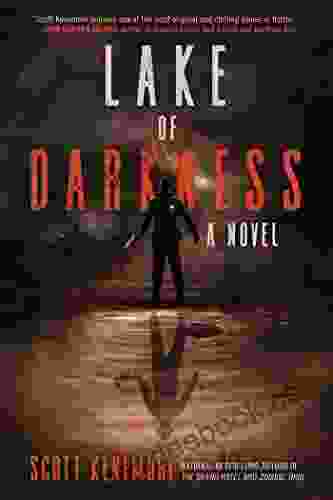

Unveiling the Secrets of the Lake of Darkness Novel: A...
A Journey into Darkness...
4.1 out of 5
Language | : | English |
File size | : | 19568 KB |
Text-to-Speech | : | Enabled |
Screen Reader | : | Supported |
Enhanced typesetting | : | Enabled |
Print length | : | 811 pages |