Hate Speech Detection and Sentiment Analysis: A Comprehensive Guide using Machine Learning and Deep Learning

In the digital age, online communication has become increasingly ubiquitous. However, this has also given rise to the proliferation of hate speech and harmful content, which can have severe consequences for individuals and society. Hate speech incites hatred, violence, or discrimination against individuals based on their race, ethnicity, religion, gender, sexual orientation, or disability. It can create a hostile environment, silence marginalized voices, and undermine the fabric of our communities.
4.6 out of 5
Language | : | English |
File size | : | 5502 KB |
Text-to-Speech | : | Enabled |
Enhanced typesetting | : | Enabled |
Print length | : | 221 pages |
Lending | : | Enabled |
Screen Reader | : | Supported |
Sentiment analysis, on the other hand, is the process of determining and categorizing the emotional tone of a piece of text. It involves identifying the sentiments expressed in the text, whether positive, negative, or neutral. Sentiment analysis is widely used in various applications, such as social media monitoring, customer feedback analysis, and product reviews.
Machine learning and deep learning techniques have proven to be powerful tools for both hate speech detection and sentiment analysis. These techniques allow us to automatically analyze large volumes of text data, identify patterns and make predictions. In this article, we will provide a comprehensive guide to hate speech detection and sentiment analysis using machine learning and deep learning, covering the following aspects:
- Data Preprocessing
- Feature Engineering
- Model Selection
- Evaluation Metrics
- Real-World Examples and Case Studies
2. Data Preprocessing
The first step in any machine learning or deep learning project is data preprocessing. This involves preparing the data for analysis by cleaning, transforming, and normalizing it. For hate speech detection and sentiment analysis, data preprocessing typically includes the following steps:
- Data Cleaning: Removing noise, duplicate data, and irrelevant information.
- Tokenization: Splitting the text into individual words or tokens.
- Stemming: Reducing words to their root form to improve model performance.
- Lemmatization: Similar to stemming, but considers the context of the word to preserve its meaning.
- Stop Word Removal: Removing common words that do not contribute to the meaning of the text.
- Normalization: Converting all text to lowercase, removing punctuation, and standardizing spelling.
3. Feature Engineering
Once the data is preprocessed, the next step is feature engineering. This involves extracting meaningful features from the text that can be used by the machine learning or deep learning model. For hate speech detection and sentiment analysis, common features include:
- Word Frequency: The number of occurrences of each word in the text.
4.6 out of 5
Language | : | English |
File size | : | 5502 KB |
Text-to-Speech | : | Enabled |
Enhanced typesetting | : | Enabled |
Print length | : | 221 pages |
Lending | : | Enabled |
Screen Reader | : | Supported |
Do you want to contribute by writing guest posts on this blog?
Please contact us and send us a resume of previous articles that you have written.
Novel
Page
Chapter
Text
Story
E-book
Newspaper
Sentence
Bookmark
Glossary
Bibliography
Synopsis
Annotation
Manuscript
Scroll
Tome
Library card
Narrative
Biography
Autobiography
Reference
Thesaurus
Character
Resolution
Catalog
Card Catalog
Borrowing
Archives
Research
Lending
Academic
Journals
Special Collections
Literacy
Study Group
Thesis
Reading List
Book Club
Theory
Textbooks
Ken T Seth
Martha Freeman
H H Lee
Alta H Mabin
Eric Berkowitz
Mary Renault
Bill Adler
Leo Hunt
Helen Yendall
Matt Beighton
Dominique Wolf
James Quinn
Anthony Hamilton
Nick Rosaci
Andrea Turner Moffitt
P C Zick
Fernando F Segovia
Joe Larson
Stone Marshall
Richard Donald
Light bulbAdvertise smarter! Our strategic ad space ensures maximum exposure. Reserve your spot today!
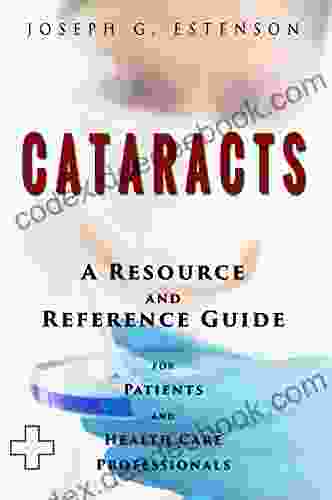

- Clark BellFollow ·3.1k
- Gabriel Garcia MarquezFollow ·11.5k
- Ivan CoxFollow ·11.7k
- Edward BellFollow ·5.3k
- Gabriel BlairFollow ·18.1k
- Eliot FosterFollow ·3.5k
- Ibrahim BlairFollow ·4.7k
- Jerry WardFollow ·9.3k
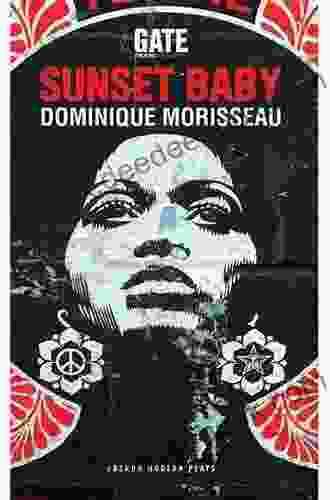

Sunset Baby Oberon: A Riveting Exploration of Modern...
In the realm of...
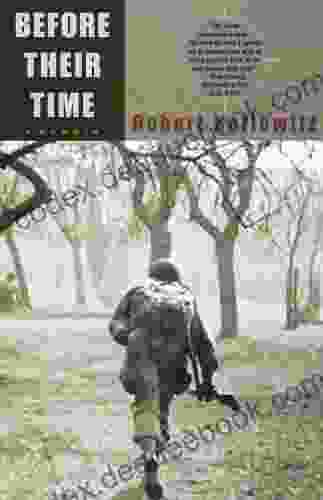

Before Their Time: A Memoir of Loss and Hope for Parents...
Losing a child is a tragedy...
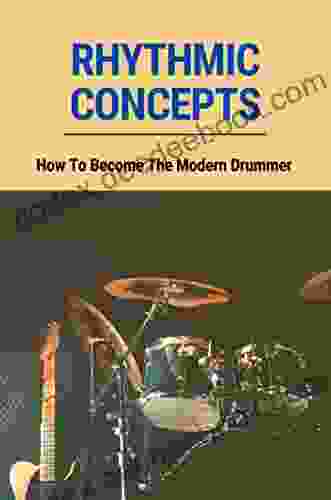

Rhythmic Concepts: How to Become the Modern Drummer
In the ever-evolving...
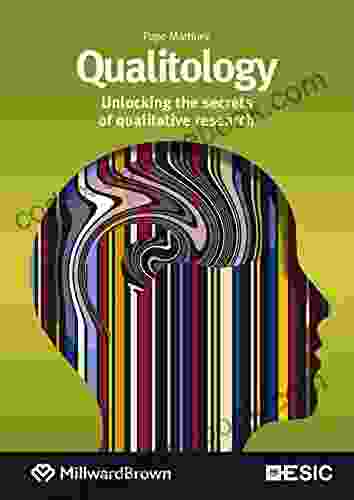

Qualitology: Unlocking the Secrets of Qualitative...
Qualitative research is a...
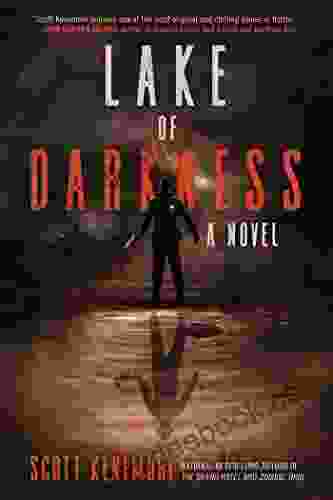

Unveiling the Secrets of the Lake of Darkness Novel: A...
A Journey into Darkness...
4.6 out of 5
Language | : | English |
File size | : | 5502 KB |
Text-to-Speech | : | Enabled |
Enhanced typesetting | : | Enabled |
Print length | : | 221 pages |
Lending | : | Enabled |
Screen Reader | : | Supported |